The unproven promise of the 80/20 rule
Lifetime value (LTV) has had a massive promise for a long time. The 80/20 rule is well understood by most consumer companies: 80% of revenue comes from 20% of your customers - called high value (high LTV) customers. This means that the LTV rate – the rate of high LTV vs. regular customers is 20/80 for this company.
LTV Rate = High LTV customers/ Regular customers
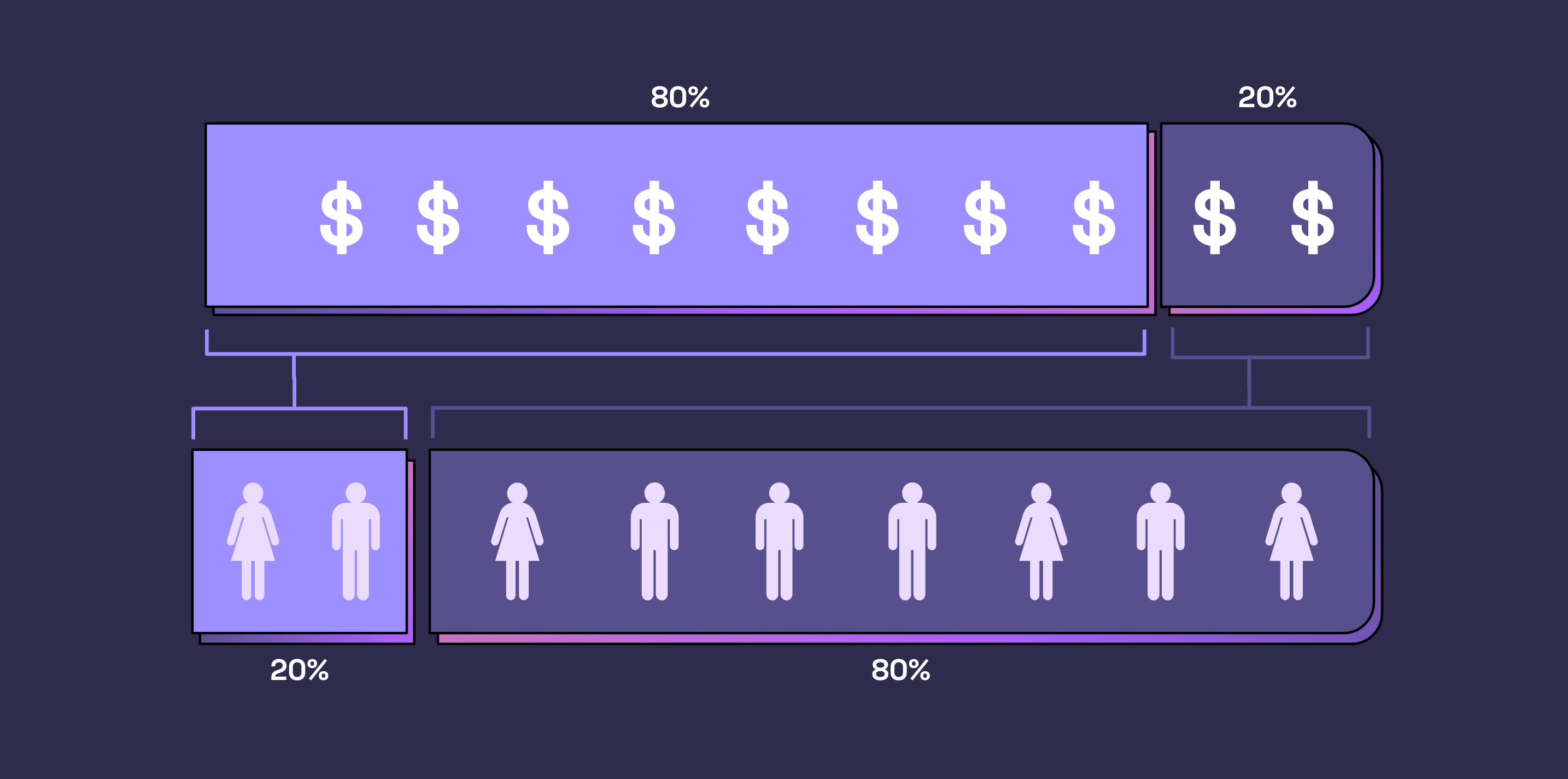
Example: The 80/20 Rule
Increasing that LTV rate (Regular/High LTV customers) ever so slightly has massive business impact. The rate can be improved by
1. Adding high LTV customers
2. Turning existing regular customers into high LTV customers
Example: Assume company X for which the best 20% of customers generate
80% of revenue has an LTV rate of 20 (high LTV customers)/80 (Regular customers). If the company manages to increase the LTV rate to (25/75) for
the next 100 customers acquired, and moves 5% of its existing customers into
the High LTV bucket, the automatic increase in revenue will be 17% compared
to the status quo approach, i.e. just adding 100 customers, 20 of them high
LTV customers and 80 of them regular customers.
This is especially relevant in times of uncertainty: macroeconomic trends paired with a political and technological privacy revolution, paired with consumer skepticism means that (internet) consumer companies are more pressed than ever to find alternative sustainable growth strategies, especially those built around their own data.
But the 80/20 rule is challenge and opportunity at the same time. The concept of the LTV shift promises massive, sustained revenue gains, but the shortcomings of AI-based solutions are obvious, and repeatedly materialized over the years: How can predicted LTV be applied to achieve this rate shift? In other words, how can predictive LTV be made actionable?
The answer: Realtime LTV.
What is Realtime LTV?
What we mean by Realtime LTV is best understood through a customer story: Imagine two shoppers accessing a website of a hair dying product company for the first time.
Hayley buys a hair pigment cream for pink hair coloring (tint rinse) for a one-time costume party – she does not have gray hair. Her LTV is low and can’t be influenced. In other words, Hayley’s LTV will be exactly the same, no matter how the brand treats her, or what experience the business curates for her.
Martha buys the same tint rinse because she has experienced graying recently and gotten self-conscious about it. To the brand, both customers look exactly the same after the first, initial purchase. But, Hayley is a one-and-done customer who will never come back, and Martha is a high potential revenue customer.

Example: Not all customers are created equal
Martha has a proverbial dollar sign over her head – her spending potential. While Hayley’s revenue potential never changes, her dollar sign changes all the time, with every touchpoint with the brand.
She likes the landing page because it provides educational content, and the dollar sign goes up. In between her site visits, she is emailed a “fire sale discount” – she hates this because she is a sophisticated gal, and her dollar sign drops.
Before the next visit to the site, she is emailed generic product information that adds little value – the dollar sign goes down further. One day later, she purchases. The dollar sign goes up to reflect the purchase, but immediately after, she is emailed aggressive discounts not related to the product, and her revenue potential yet again plummets, this time to zero.

Example: Current customer journey of high LTV customer
In short, from the first interaction with the educational content, every touchpoint has the ability to increase or decrease this buyer’s spending potential. That is exactly what we coin as Realtime LTV.
”Realtime LTV is the ability to predict the future revenue of a customer, and adjust the prediction based on every customer touchpoint with the brand.”
Realtime LTV can differentiate between Hayley and Martha immediately. And Realtime LTV allows brands to turn LTV into a benchmark against which transparent experimentation (down-funnel) and acquisition (up-funnel) strategies can be built that achieves exactly that: Change the LTV rate by acquiring more high LTV customers and pushing more existing customers into the high LTV bucket – more on that further below. But first, there is a big if: How can Realtime LTV be obtained?
Revolutionary technology for Realtime LTV
Realtime LTV has been a significant product challenge. Until this approach and to our knowledge, there has been no solution solving the Realtime LTV challenge.
Right from the start, we can break down Realtime LTV into two necessary components:
1. High-fidelity data (read: massive time-granular user data)
2. Sophisticated Machine Learning that can make sense of this data and apply it to LTV.
Cracking Realtime LTV means cracking both components, it is an engineering challenge as much as a Machine Learning challenge. This is how we have done it:
Data accelerating LTV to Realtime
In order to solve for Realtime LTV, additional data needs to be leveraged. We have explored most classes of data under the sun – everything from large-scale administration data we have incorporated into predictive AI for years, to credit-card panel data, to IRI consumer data.
None of these data sources had the right attributes of high-fidelity. And, linkage between these data sources will increasingly depend on the willingness of Big Tech players (such as Google), and come under regulatory scrutiny fast.
Our approach instead: Cloud-based technology surfaces curated streams of behavioral consumer data related to LTV for each brand. This data is gathered by a proprietary piece of software, which is deployed as a website pixel.
The software collects precise details of users' shopping behavior based on event and click stream data (e.g. time between add-to-cart and checkout, products viewed, time spent on site, etc.), pairs it with transactional/purchase and engagement data sourced through API integrations and then iteratively goes back and forth between the ML algorithm and the software to hone in on a profile of event stream data significantly related to LTV.
This initial learning takes 60-90 days (but continues over time), after which the resulting curated/brand-specific profile of event stream data accelerates LTV predictions to Realtime.
ML accelerating LTV to Realtime
The underlying proprietary machine learning algorithm builds on a class of structured probabilistic models called Buy-Till-you-Die, that model customer purchase patterns as well as churn (in this language called: death) as distributions and adds a deep learning/neural nets component along with model explainability, allowing machines to make sense of it all.
That means uncovering relations between the massive behavioral data we leverage for each prospect and customer, and LTV. The algorithm has been validated against actual purchase data, and routinely achieves above 90% accuracy.

Example: Data and AI powering Realtime LTV
Applications of Realtime LTV
Applications of Realtime LTV span two areas
1. Realtime LTV for customer acquisition
2. Realtime LTV for optimization
Realtime LTV for acquisition
Given the tectonic shifts to attribution and the closed “Walled Garden'' nature of most acquisition channels/platforms (read: data in, no data out), trying to manipulate or improve results by pulling some of the few levers available to the marketer on these platforms is a fool’s errand.
Instead, sophisticated brands try a number of acquisition strategies – maybe TikTok, Meta, Alphabet and influencers – and measure performance dynamically so that the channel mix can be optimized.
Problem is, evaluating acquisition channels or campaigns based on performance is hard. Think back to the two customers – the jokester and the serious shopper – from above. Both customers purchase the same item, but we don’t want to count both events equally.
Better approaches rely on predictive LTV, but this requires a number of purchases for accurate predictions. Based on the length of the sales cycle, brands end up evaluating the Metas and Alphabets of four or so months ago – which have little to do with the performance of these channels today.
Realtime LTV helps brands understand – week by week, day by day – the Realtime revenue potential generated by each channel or ad campaign, and enables efficient redistribution of resources at a regular cadence. Realtime LTV helps brands understand – week by week, day by day – the real time revenue potential generated by each channel or ad campaign, and enables efficient redistribution of resources efficiently at a regular cadence.
This is expressed in the example below – we have seen many times that channels yield a better Cost-per-Acquisition (CPA), but a lower first-year LTV. Any data-driven marketer will want to scale spend on Google, but Realtime LTV is needed to make this (correct) decision.
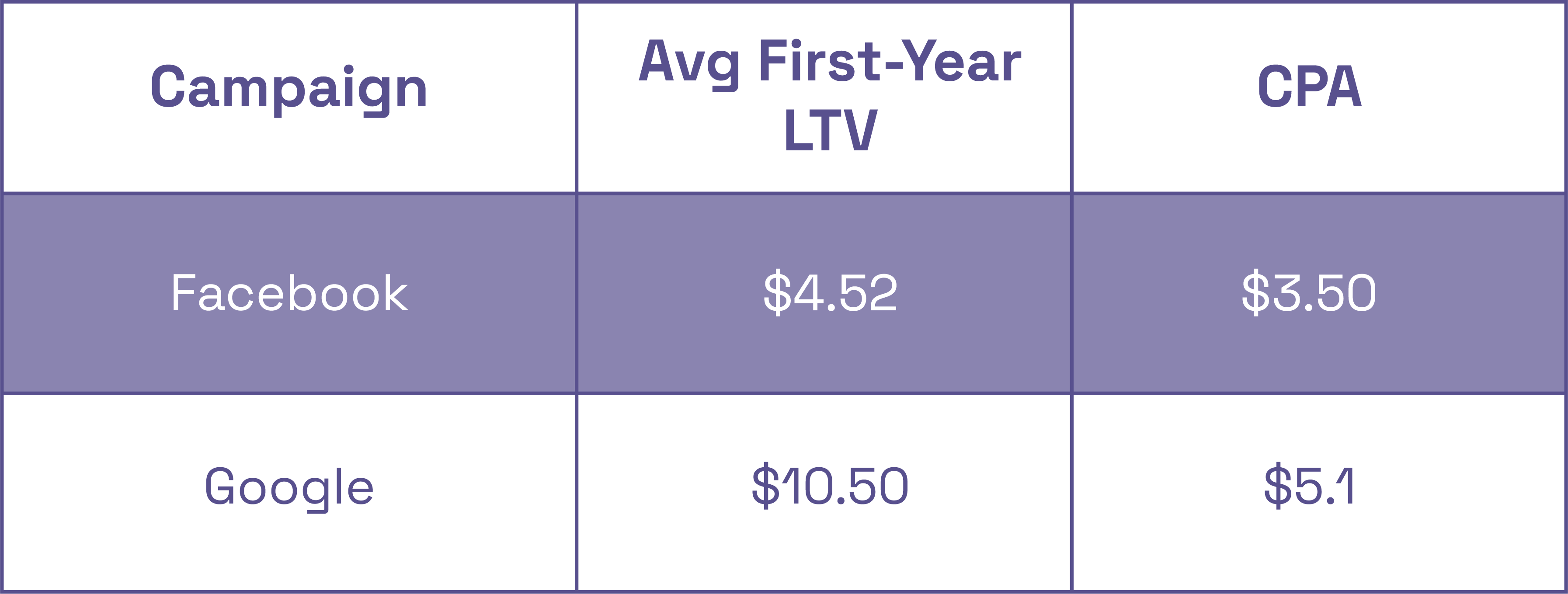
Realtime LTV for optimization
If Realtime LTV is known, it can be used as a benchmark for every A/B test brands run. Realtime LTV allows consumer companies to test over the right high-value customers in shorter cycles – because every movement to revenue potential is now picked up – on metrics that matter most, i.e. predicted revenue.
Based on our experience, using Realtime LTV as the evaluation/measurement for experiments means that experiments can be wrapped up in less than two weeks and require a much smaller sample to be sufficiently powered, i.e. uncover true effects reliably. It increases efficiency all around.
If Realtime LTV is integrated into major testing platforms, brands can optimize all testing on Realtime LTV through their existing work-flow with no lift.

Example: Realtime LTV used to evaluate 3 Treatment conditions
Tests can run across engagement, pricing, product specs, user experience and more.
And, a testing framework based on Realtime LTV means internet consumer companies can get from the reality above to a customer experience optimized for high-value customers, one in which every touchpoint – the landing page, the discount, the engagement – is tailored to this group of customers with high revenue potential, such as Martha who is genuinely in the market of becoming a high value customer.
Our experiments have shown that optimizing even small parts of the customer journey can increase LTV of the most valuable customers by 12%. For any 80/20 company, this will increase the revenues by north of 12%.
Ultimately, brands get to build their custom growth playbook by
(1) optimizing acquisition against Realtime LTV
(2) experimenting against Realtime LTV efficiently and fast
That is the power of
Realtime LTV.